How fast are LLMs in production?
At Straive, we use an LLM Router. Since ChatGPT, etc. are blocked for most people, this is the main way to access LLMs.
One thing we measure is the speed of models, i.e. output tokens per second. Fast models deliver a much smoother experience for users.
This is a different methodology than ArtificialAnalysis.ai. I’m not looking purely at the generation time but the total time (including making the connection and the initial wait time) for all successful requests. So, if the provider is having a slow day or is slowing down responses, these numbers will be different.
Hopefully this gives you a realistic sense of speed in a production environment.
Here’s the speed of models with at least 500 requests over the last 2 weeks. I’ve grouped the models based on speed grades
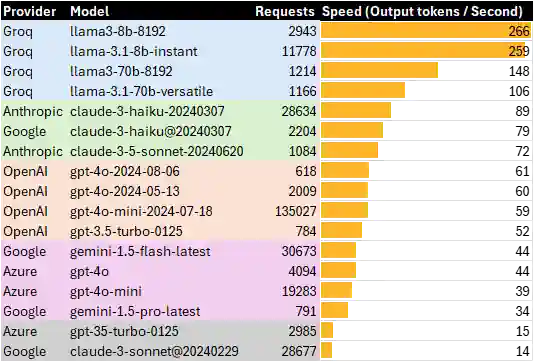
Grade 1: 100+ Tokens / second. Groq is clearly serving the Llama 3 models at blazing speed. No surprises there — except why Groq still doesn’t let me pay. The free tier is open with generous rate limits and the Pay per Token model has been “Coming Soon” for several months now (and I’ve no complaints 🙂).
Grade 2: 70+ Tokens / second. Anthropic’s Claude 3 Haiku is the next fastest class of models, but Claude 3.5 Sonnet is surprisingly fast, almost as fast as Haiku and over 70 tokens per second. This is impressive.
Grade 3: 50-60 Tokens / second. OpenAI’s GPT 4o models are almost as fast. It’s interesting that GPT 4o and GPT 4o mini are at about the same speed! GPT 3.5 Turbo is not far behind either. Perhaps OpenAI increases capacity for slower models?
Grade 4: 30-50 Tokens / second. Gemini 1.5 Flash is a much, much slower than the benchmarks – maybe we’re doing something wrong. Azure’s GPT 4o service is about twice as slow as OpenAI’s, and comparable is speed with Gemini 1.5 Pro.
Grade 5: <20 Tokens / second. Azure’s GPT 3.5 Turbo and Google’s Claude 3 Sonnet are among the slowest ones. These are older models on third-party infrastructure, so I suspect they’ve been given weaker infrastructure (unlike OpenAI which is serving GPT 3.5 Turbo at 3X the speed Azure does.)
Drivers of speed
Here’s what I’m taking away (informally):
- GPU architecture is the biggest driver of speed. Groq is FAST! Hopefully, the fact that they won’t let us pay isn’t a red flag that the service will vanish.
- How companies operate seems the next biggest driver. Anthropic’s models are consistently faster than OpenAI’s which are faster than Google’s.
- Companies run their own models faster than cloud providers. OpenAI is faster than Azure, and Anthropic is faster than Google for the same models.